Machine Learning-based Prediction of Surplus Material in Intelligent Production Processes
Keywords:
surplus material prediction, industrial data analysis, feature selection, data-driven methods, intelligent steel production systemAbstract
Industry 4.0 technologies have driven traditional manufacturing to intelligent manufacturing. With massive production data, machine-learning-based methods have been widely used to predict and control a production process intelligently. They can help manufacturers achieve efficiency improvement and cost reduction. In this work, we present a data-driven method to predict surplus materials in a cold rolling process in intelligent steel production systems. A cold-rolled steel coil is a common kind of steel products that is easy to generate surplus materials during its production. Avoiding or reducing the generation of surplus materials is desired for steel enterprises since it seriously affects their profits. However, its complex production processes make it difficult to identify the causes of surplus materials. The issue of predicting surplus material has not yet been well-addressed. In this work, a surplus material prediction problem is first proposed and solved based on a combination of statistical analysis and data-driven methods. We aim to find the key parameters that cause the generation of surplus material and predict whether surplus materials would be generated during a production process. Based on an industrial dataset, several machine learning methods are developed to build a prediction model that is able to meet actual requirements of steel coil production processes. The experimental results show that, among them, extreme gradient boosting and logistic regression methods are highly reliable with the best performance. In addition, an explicit expression obtained by logistic regression can provide practitioners with excellent guidance in their practical applications.
Downloads
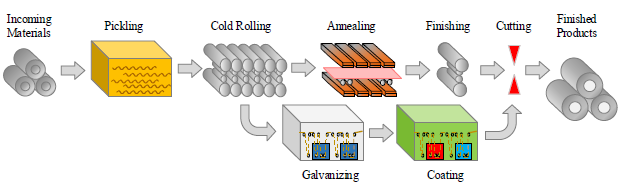
Downloads
Published
License
Copyright (c) 2025 International Journal of Artificial Intelligence and Green Manufacturing

This work is licensed under a Creative Commons Attribution 4.0 International License.