A Partition Stacking Classification Framework With Oversampling for Quality Prediction of Aluminum Alloy Ingots
Keywords:
Aluminum alloy ingot, Quality prediction, Stacking, Polynomial Fitting SMOTE, Data partitionAbstract
As the raw material of aluminum alloy production, the quality of aluminum alloy ingots has an important influence on the quality of final products. We study how to use machine learning models to predict ingot quality by production parameters. However, traditional machine learning models often ignore upstream and downstream relationships and the data imbalance in practical production. In this paper, to solve the above problems, knowledge about the aluminum alloy melting-casting process is applied and the relationship between process parameters of melting-casting process and ingots quality is modeled via a partition classification framework based on standard stacking. The data is divided into three parts according to different processes, and then sequentially input the framework in process order. The major difference between our method and the standard stacking is that, at the base-level, the classifiers' predictions for each part are fused with the next part as the new input. After that, the output of the last part is then fused with all the data for training at the meta-level. We carefully compare the performance of Synthetic Minority Oversampling Technique (SMOTE) variants, and an oversampling method called Polynomial Fitting SMOTE is used in the classification framework to deal with the imbalance problem. We apply our proposed method and obtain the best classification results on aluminum alloy melting-casting data. We also show the validity of the combination of Polynomial Fitting SMOTE and standard stacking on public datasets.
Downloads
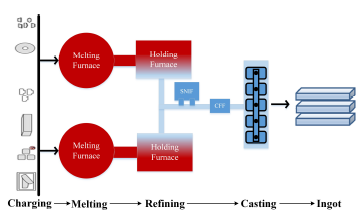
Downloads
Published
License
Copyright (c) 2025 International Journal of Artificial Intelligence and Green Manufacturing

This work is licensed under a Creative Commons Attribution 4.0 International License.